Category: Education
Colleges (0)
UK (0)
Universities (0)
Nicole Grace Blog Open the blog
Latest Blog Posts
How to Remove Damaging Content from Internet Search Results
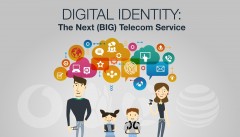
best eleven world cup 2018, play football game on instantfuns Open the blog
BEST ELEVEN: WORLD CUP 2018 is the first ever football manager game on the H5 platform. The game simulates the entire football game through an independently developed AI engine.
Our Management Approach Favours Teamwork Open the blog
To meet our clients’ long-term wealth management objectives, we implement a conviction based management approach within a streamlined management team and a deliberately narrow range of funds. Our fund managers are experts in their respective fields. Each morning, they exchange views and site visit reports in order to develop their investment strategy.
Our principles
Latest Blog Posts
Our Management Approach Favours Teamwork
Our Management Approach Favours Teamwork
Software Training and Job Placement Open the blog
Latest Blog Posts
Iot Training in Bangalore Open the blog
IOT Training in Bangalore - Live Online & Classroom
IOT Training course observes iot as the platform for networking of different devices on the internet and their inter related communication.
Latest Blog Posts